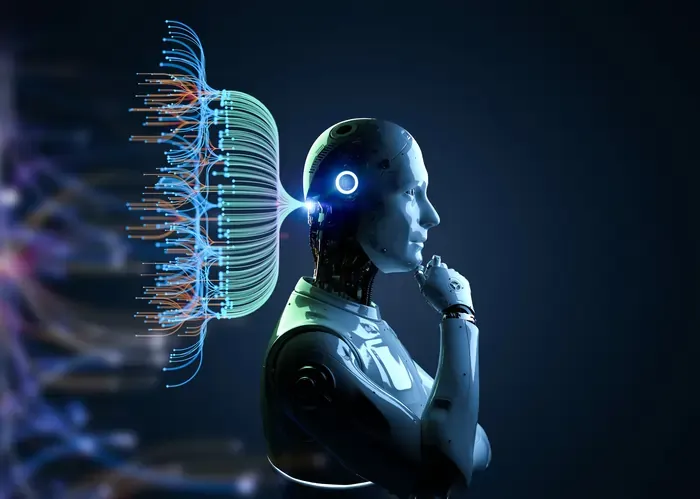
The Role of Machine Learning in Predictive Maintenance: A Comprehensive Insight
Predictive maintenance stands at the forefront of industrial innovation, thanks to the integration of machine learning technologies. This transformative approach not only promises to revolutionize the way businesses conduct maintenance but also ensures unparalleled efficiency and reliability. By harnessing the power of machine learning, companies can anticipate equipment failures before they occur, saving time, resources, and potentially millions in operational costs.
Understanding Predictive Maintenance
Before delving into the role of machine learning, it’s crucial to grasp what predictive maintenance entails. This proactive strategy uses data analysis tools and techniques to detect anomalies in equipment operation and potential failures before they happen. Unlike traditional maintenance practices, predictive maintenance offers a more targeted approach, minimizing downtime and extending the lifespan of machinery.
Machine Learning: The Driving Force Behind Predictive Maintenance
Machine learning, a subset of artificial intelligence, empowers predictive maintenance with its ability to learn from data, identify patterns, and make informed predictions without human intervention. This section explores how machine learning algorithms are the linchpin in predictive maintenance strategies, transforming raw data into actionable insights.
Predictive Maintenance in Action: Real-World Applications
The application of machine learning in predictive maintenance spans various industries, from manufacturing to energy. This section will showcase several case studies where predictive maintenance has significantly reduced costs, improved safety, and enhanced operational efficiency, illustrating the tangible benefits of this technology.
The Benefits of Machine Learning-Driven Predictive Maintenance
The advantages of integrating machine learning into predictive maintenance are manifold. This segment will dissect the key benefits, including reduced operational costs, decreased downtime, and improved equipment lifespan, providing a compelling case for businesses to adopt this innovative approach.
Challenges and Considerations
Despite its numerous advantages, implementing machine learning-driven predictive maintenance is not without its challenges. This part will address the potential hurdles businesses may face, such as data quality issues, the need for skilled personnel, and the initial investment required for technology adoption.
Future Trends: Where Machine Learning and Predictive Maintenance Are Headed
The future of predictive maintenance, fueled by advancements in machine learning, looks promising. This section will speculate on upcoming trends, such as the integration of IoT devices and the use of more sophisticated algorithms, outlining how these developments could further enhance predictive maintenance strategies.
How to Implement Machine Learning in Your Predictive Maintenance Strategy
Implementing machine learning into predictive maintenance requires careful planning and execution. This guide will provide step-by-step advice on how to start, from selecting the right tools and technologies to training your team and analyzing data effectively.
Case Studies: Success Stories of Predictive Maintenance
Highlighting real-life examples, this section will delve into successful implementations of predictive maintenance across different sectors. These case studies will demonstrate how machine learning has enabled companies to foresee and prevent equipment failures, underscoring the practical value of this approach.
The Role of Data in Machine Learning and Predictive Maintenance
Data is the lifeblood of machine learning and, by extension, predictive maintenance. This segment will explore the importance of high-quality data, how to collect and process it, and the role it plays in making accurate predictions.
Machine Learning Algorithms for Predictive Maintenance
An overview of the specific machine learning algorithms commonly used in predictive maintenance, including regression analysis, neural networks, and decision trees. Understanding these algorithms is crucial for grasping how predictive maintenance models are built and function.
Integrating Predictive Maintenance into Existing Systems
For many businesses, the challenge lies not just in adopting predictive maintenance, but integrating it seamlessly with existing operations. This section will offer insights into how companies can merge new predictive maintenance strategies with their current processes without disrupting workflow.
The Economic Impact of Predictive Maintenance
Examining the broader economic implications of predictive maintenance, this part will discuss how this approach can lead to significant savings for businesses, contribute to environmental sustainability, and even impact the global economy by reducing waste and increasing efficiency.
Predictive Maintenance and Industry 4.0
Predictive maintenance is a cornerstone of Industry 4.0, the new era of automation and data exchange in manufacturing technologies. This section will contextualize predictive maintenance within the larger framework of Industry 4.0, illustrating its role in advancing smart factories and connected manufacturing.
Ethical Considerations in Machine Learning for Predictive Maintenance
As with any technology that leverages data, there are ethical considerations to be mindful of. This segment will address privacy concerns, data security, and the responsibility of businesses to use predictive maintenance technologies ethically and transparently.
The Intersection of Machine Learning and IoT in Predictive Maintenance
The Internet of Things (IoT) plays a pivotal role in enabling effective predictive maintenance. This part will explore how IoT devices collect the crucial data that machine learning algorithms analyze, highlighting the symbiotic relationship between these technologies.
Overcoming Data Silos for Effective Predictive Maintenance
Data silos can hinder the effectiveness of predictive maintenance programs. This section will discuss strategies for breaking down these barriers, ensuring that data flows freely across different departments and systems, thereby enhancing the accuracy of machine learning predictions.
Skills and Training for Predictive Maintenance Teams
With the technical nature of machine learning and predictive maintenance, having a skilled team is essential. This segment will cover the types of skills needed, how to build or upskill your team, and the importance of continuous learning in this rapidly evolving field.
Measuring the Success of Predictive Maintenance Initiatives
Implementing predictive maintenance is one thing, but measuring its success is another. This section will provide metrics and KPIs that businesses can use to assess the effectiveness of their predictive maintenance efforts, ensuring they achieve their desired outcomes.
Predictive Maintenance Tools and Software
A review of the leading tools and software solutions available for predictive maintenance. This part will help businesses navigate the crowded marketplace, offering insights into selecting the right tools that align with their specific needs and objectives.
The Synergy Between Predictive Maintenance and Sustainable Practices
Predictive maintenance not only boosts operational efficiency but also promotes sustainability. This section will delve into how predictive maintenance practices can reduce waste, lower energy consumption, and contribute to a more sustainable future.
Frequently Asked Questions
- What differentiates predictive maintenance from traditional maintenance?
- How does machine learning improve the accuracy of predictive maintenance?
- What industries benefit most from predictive maintenance?
- Can small businesses implement predictive maintenance effectively?
- How long does it take to see ROI from predictive maintenance initiatives?
Conclusion
Machine learning in predictive maintenance is not just a trend but a fundamental shift in how businesses approach equipment maintenance. By leveraging data and advanced algorithms, companies can anticipate failures, optimize operations, and achieve significant cost savings. As technology advances, the role of machine learning in predictive maintenance will only grow, offering even greater opportunities for efficiency and innovation.