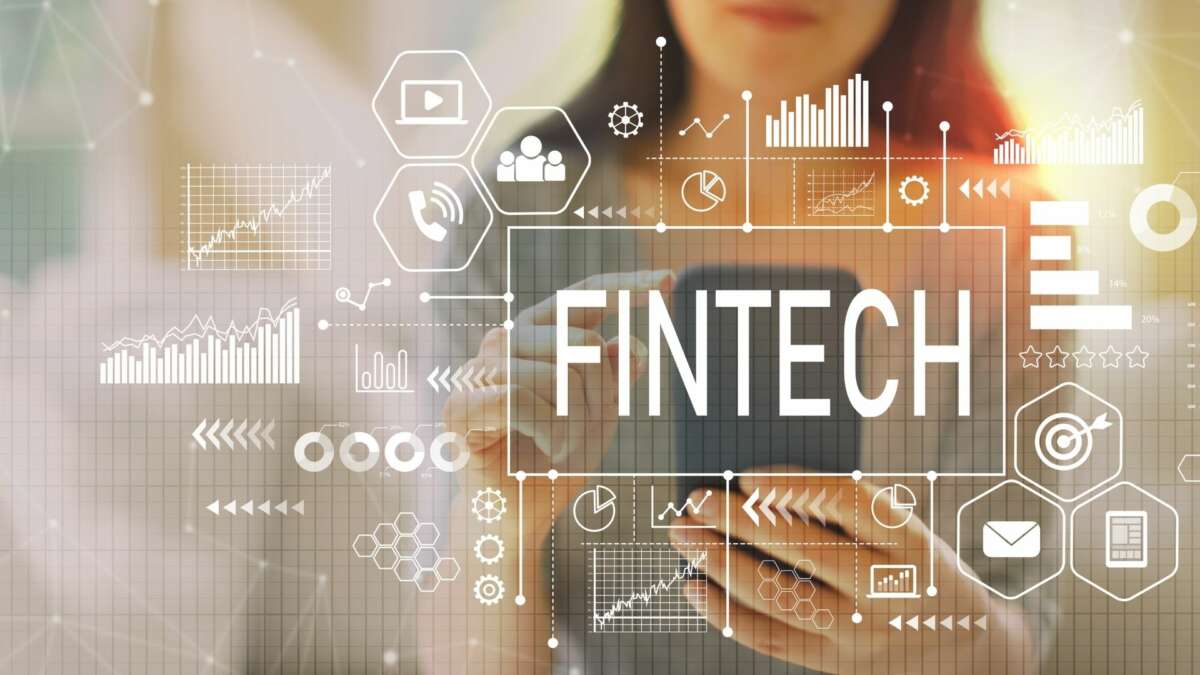
By Christopher Warhurst,Technical Director, 4most
Fintech lenders aim to challenge and disrupt the standard lending model offered by high street banks through greater user experience, often achieved with smartly designed apps and more efficient processes. An example of this is reducing the number of clicks needed to open accounts, thereby removing friction and effort by using similar data that is already available, albeit with the potential for nuance to be lost. Key aspects of the business model are, being able to develop and launch products and services quickly, easily andmore cost effectively, while being designed with single-mindedness to what the customer needs. To facilitate this, the organisations are often lean in structure, enabling quicker decisions and access to funds, especially in relation to overheads that are seen as bureaucracy or blockages to agility and speed.
The increased use of models in place of human judgement has been a key part of lending practices for several decades. For example, to enable straight through decisioning on lending applications,statistical models are deployed to assess aspects of the borrower for their credit worthiness (including affordability), fraud and financial crime. This allows rulesets and algorithms to be applied and the potential for a loan to be approved without the need for human intervention.However, whilst traditional banks’ processes will often have elements of manual intervention, such as for marginal risk cases or affordability confirmation, fintechs will often place much greater confidence in models to perform these tasks without intervention.In keeping with the aim to provide an improved user experience, the result on operational effectiveness alone is clear with somefintechs having automated decisions rates well in excess of 90%, even for complex products such as secured lending, whereas traditional banking models would manually underwrite the large majority of decisions.
Responsible lending practices
This level of automation results in anincreased reliance being placed on the models with regards to the profitability and robustness of the subsequent portfolio. In addition, from a regulatory perspective, all lenders are regulated by the Financial Conduct Authority (FCA) and therefore need to ensure that they are fulfilling expectations and treating customers fairly, both in terms of discrimination and responsible lending practices. This is an area that will become even more stringent following the introduction of the consumer duty.
Furthermore, once on the balance sheet, firms are required to continually assess the risk and quality of their loans for use in accounting (IFRS 9 Expected Credit Loss) and Internal Rating Based (IRB) models for deposit takers wishing to make more effective use of capital supply. All of the requirements and impacts can result in a reliance across a number of models with significant implications for the business,in terms of profitability, stability, robustness and regulatory compliance should one or more ‘fail’.
Current approaches to model risk
This in turn leads to the creation of expansive and complex model risk within the organisation. But what is model risk, how is it mitigated,do fintech’s approach it any differently to the rest of the banking industry and if not, should they?
Model risk relates to the risk incurred across a range of areas as a result of its reliance on models in the operation of the organisation. This risk can exist due to a number of sources including, but not limited to, the model not performing as expected, the model not being used in the correct manner, the model not being developed correctly, the model not being appropriately maintained, corruption of the data feeding the model, or inaccuracies in its implementation. Should one of these issues occur, the realisation of the risks can manifest themselves financially, reputationally or through compliance issues.
The mitigation of these risks is through strong internal governance, ongoing monitoring and good understanding and direction from the top of the organisation i.e., the board. Within the UK the Prudential Regulation Authority (PRA) are beginning to provide more direction with the publication of Consultation Paper 6/22 – Model Risk Management Principles for Banks. This consultation paper outlines the expectations regarding having named senior management responsible for model risk, the scope of model risk, the need to potentially include material non-models, and the definition of materiality alongside its need to consider complexity.
The current approach to model risk within UK financial services is at varying levels of maturity. For larger established organisations, particularly those with IRB accreditation, model risk has been developing over a number of years and these are generally well-established frameworks including detailed model performance reporting, well-defined model governance routes and associated policies, independent validation functions and executive level reporting material containing key performance indicators and associated triggers. However, there is still further work to be done based on the consultation paper, with specific comments being made regarding “areas such as board involvement and understanding”.
The burden on board members to gain and maintain the technical knowledge required to thoroughly understand the risks, validity and limitations of models is high and is critical when models are possibly the most significant tool in the running of the organisation, as they often are in fintech lenders. There is a danger that non-technical specialists can rely on models being objective and infallible and therefore outside of the board’s area of consideration and direction. However, as is well understood in decision science functions, there is a large amount of subjectivity and limitations within models that,if not understood at the top level, can lead to what in effect becomes a high level of delegation of key aspects of the business and the subsequent creation of undetected model risk.
Machine learning models
Another interesting component of CP 6/22is the need to consider complexity in the materiality assessment of a model.This highlights that even if a model is not necessarily considered to be material in the role it plays, it should be considered more material if it is of a complex nature. This immediately raises the consideration of machine learning models and the requirement to automatically consider them as a higher material model than their usage alone would indicate. The use of machine learning models is clearly becoming a key focus of the regulators as it has been highlighted as a key reason for the need to increase model risk management capability as it “anticipates firms’ use of models will continue to increase and become more complex as new model types are introduced”.
For finetchs the usage of machine learning models is seen as a potential key differentiator in allowing greater reliance on automation and the leveraging of more agile data systems, that are not burdened with the legacy issues inherent in long-established high-street lenders. This is highlighted in a 2019 FCA paper regarding the role of machine learning in UK Finance Services “Hence, this new data-driven economy goes hand in hand with fundamental changes to the structure and nature of the financial system supporting it. And ML is a principal driver contributing to this new finance.”This should be seen by fintechs as an invitation to disrupt the financial services market to offer greater breadth of products, competition and customer service to the industry and to use advanced models to achieve this.
However, as already highlighted, there is a higher bar for more complex models that does make sense.With more complex models the range and amplification of model risk should be seen as greater due to the lower transparency and increased reliance on algorithms, as opposed to human intervention. An example ofthis risk was highlighted in 2019 with the publicity regarding the Apple card allocation of credit limits and its supposed bias against women. It should be noted that this complaint was not ultimately upheld due to the omission of gender, or any directly related variable, from the model. However, there was widespread negative publicity and numerous comments made regarding how much certainty there was that complex interactions within the model were not proxying gender.
Deterioration in model performance
This case highlights one potential manifestation of model risk, namely conduct and complianceconcerns inherent in more complex models.However,a likely greater manifestation of model risk will be financial risk due to poor model driven decisions. This can occur due to models not being reflective of the true likely outcomes and therefore resulting in either poor quality customers being approved, increasing bad debt, or good quality customers being declined, losing profitable business.This can occur for a number of reasons but is most commonly due to deterioration in the model performance from point of development.
Model deterioration is common and is mitigated through robust model monitoring and ongoing maintenance. A strong model monitoring process including detailed characteristic analysis, well defined performance thresholds and clear escalation mechanisms are key to a well-established model risk management process. However, this should not be seen as the only aspect, which is often an assumption thatis made in the development of less established model risk frameworks.
Model monitoring only gives an indication of how well the model has predicted reality in the recent past but in times of large change and uncertainty,this is not sufficient to protect against poor decision making. In recent times the impact of Covid on model performance has been monumental, with both characteristics and outcomes largely distorted due to changes in behaviours, unprecedented government support and key economic indicators reaching levels outside of anything seen previously e.g., GDP growth. During such times,had a fintech, largely reliant on automated decisioning, waited until the quantitative monitoring triggered red, they would have likely found themselves making poor business decisions for well over a year whilst the outcome data settled, and the true performance was realised. The current cost of living crisis introduces similar considerations, where it is known that certain pockets of the population will suffer greater than others and will perform worse in terms of their ability to service debt.
Forward-looking measures with a strong risk culture
Therefore, there is a need within the model risk framework to include an element of qualitative assessment of more forward-looking measures and the data that is available today. This obviously takes time, effort and resource and may not be fully aligned to an aspirational lean organisational approach, reliant upon data and models, but it is a necessity to guard against large negative impacts on business performance.
This oversight and human intervention comes back to the need to have strong senior involvement with a good risk culture up to board level (i.e., a culture that is wary of the hubris that can occur in some organisations), and also a strong second line risk function that holds to account the side of the business mandated to drive growth. In fintechs it is common to see a focus being placed on the user journey and in particular the unique selling point of the given lender – be that well designed apps, quick decisioning, product offering etc. However, one common theme for all lenders, both fintechs and established lenders, is the clearly observable benefit that a strong risk oversight throughout the organisation brings.
A well evolved risk function integrated into the running of the organisation should be seen as an enabler that allows confident expansion into new areas for the organisation. For organisations with a heavy reliance on models, this confidence comes from the function focusing on a strong model risk framework ensuring that existing model risk issues are identified through quantitative analysis and technical validation, upcoming risk issues are anticipated through human intervention and oversight, and that all of these are communicated to, and understood by, senior management, inclusive of the board.