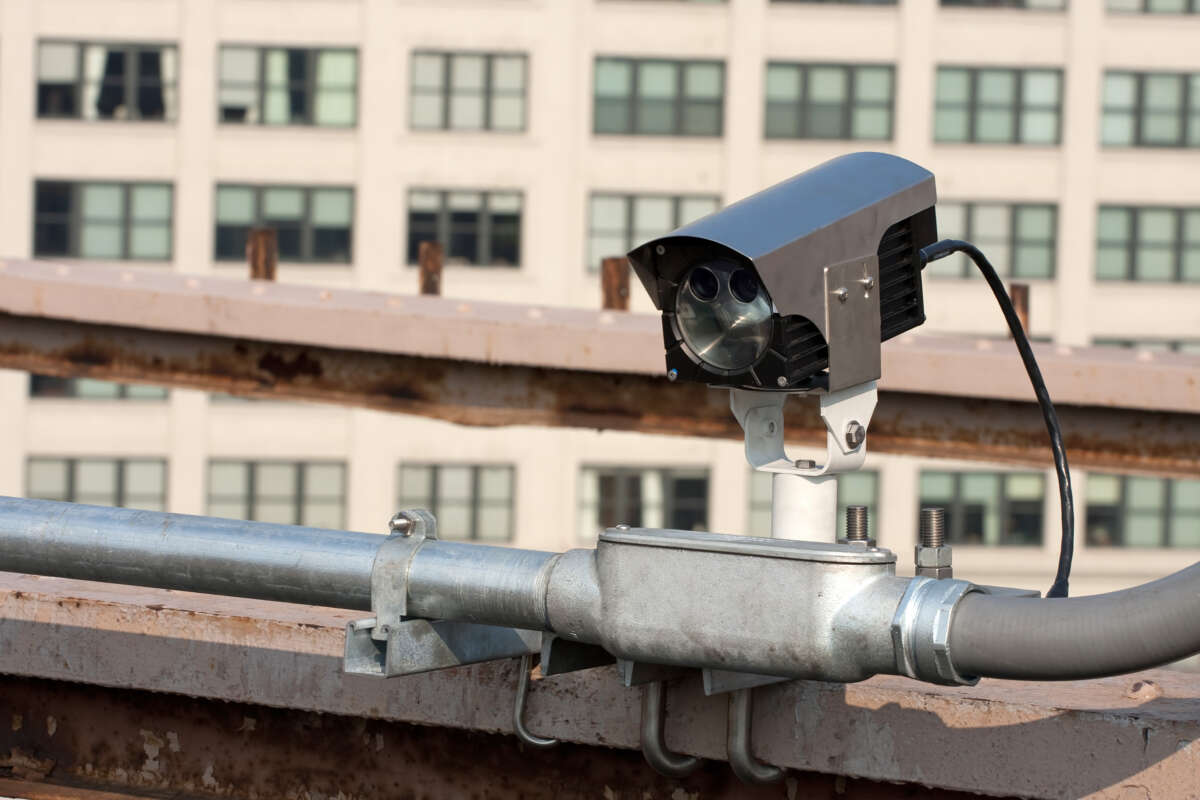
By: Paul Gibson, Business Development Director, KX
When it comes to catching potential trade market abuse and fraudulent activity, surveillance departments are under pressure from regulators in a way they have never been before. With monitoring obligations becoming increasingly complex, they’re having to rethink the way they approach identifying potential market abuse. At the same time, their analysts are increasingly weighed down by high swathes of alerts and investigations, many of which prove to be unnecessary when other factors are taken into account. It’s a double-edged sword, with businesses both needing to do more but also needing to do less.
Getting surveillance right is a deeply complex issue. Organizations often have differing surveillance solutions depending on the needs of the individual business unit, and the ongoing COVID-19 pandemic has only added further complexity. This lack of cohesion across different remote working locations means there’s also additional operational overhead and latencies associated with investigating potential breaches of compliance or malpractice. Despite these severe challenges, the regulators are not letting up on their expectations of compliance.
Regulators expect financial institutions to survey activity according to their specific business, monitoring for market abuse, fraud, market disruption and fair practice. Depending on the business model of the institution, this translates to analysis of huge amounts of both historical and real-time data. Added to this, financial institutions must ensure their clients are not using trading accounts to money launder adding further complexity to the monitoring requirements.
What’s needed is a consolidated enterprise approach to an organization’s surveillance strategy, powered by historical and real-time analysis. One that’s effective across all lines of business for detection of emergent, systemic and often unknown risk. This is key in order to proactively reduce risk and operational costs, and also capture and make sense of all of the interactions, dependencies, changes, patterns and behaviors across the entire trade lifecycle.
Firms must adopt platforms that process the vast amount of data from multiple streams in real-time, allowing users to make decisions on alerted behaviors much more effectively with significantly greater efficiency. This means using cross-product analysis to identify errors, automated techniques to reduce false positives and machine learning to extract insights from both historical and real-time data.
Analysis Cross-Product
Traditional instrument-by-instrument surveillance techniques do not typically extend their analysis to related products. This means that in certain areas, such as credit and rates, the links between the topics and how they are affecting one another go unseen. This is opposite to risk management techniques across the same technologies where trade dependencies are closely monitored.
As such, it is important to incorporate risk management elements, like benchmark and sensitivity measures to help identify potential abuse over a range of instruments. This enables products to be broken into their risk fundamentals and effectively “look through” to the underlying securities in an analysis. In looking for evidence of manipulation of a Financial Risk Advisor (FRA), for example, the analysis may extend to monitor both futures and interest rate swaps too.
Generating Less False Positives
As with cross-product analysis, having more information can lead to more insightful judgments. In the case of false positives, the presence of surrounding data can help contextualize results by automatically classifying high volumes of alerts. This helps to determine which are material and which are not. False positives reduction techniques fall into three areas:
- Data Filters – Filtering out specific data or activity that may not be applicable. For example, excluding immediate-or-cancel (IOC) orders from Spoofing profiles.
- Use of Dynamic Thresholds/Benchmarks – Replacing static thresholds with automatically adjusting parameters that reflect evolving market conditions and changing behaviors, not only of individual traders but across the market.
- Alert Feature overlays – Including surrounding factors for context in assessing alert severity. For example, factoring in change in portfolio concentration when monitoring potential insider trading.
These factors, used together, help avoid unnecessary and time-wasting alerts that distract analysts from more significant investigations. Thereby, optimizing both operational efficiencies and effectiveness for mitigation of true risks.
Why a Brighter Future Relies on Machine Learning
Machine learning allows a variety of business practices to be improved, such as calibration and error reduction. Detection rates can be continuously improved using a blend of supervised learning, unsupervised learning and feature extraction techniques from the historical data store.
Supervised learning uses analyst feedback and assessment of historical results to train models and improve their accuracy. Unsupervised learning uses techniques like One-Class Support Vector Machines (SMVs) to detect anomalies to help classify results based on distributions and similarities. SVMs establish normal behavior by learning a boundary and then adding a score to the results, based on their distance from that boundary. This adjustment can then help analysts to prioritize investigations. Whilst the benefits of AI and machine learning are well documented, their application for improving detection rates in trade surveillance remains limited.
With regulators still hesitant to allow machines to determine whether an activity is suspicious or not, the majority of what we are seeing is a supervised learning approach. However, as the regulatory landscape continues to evolve and the demand for real-time decision-making mounts, organizations will require a shift in mindset and capitalization of narrow AI with unsupervised machine learning if they are to effectively detect fraud.
It has become apparent that the requirements for strong trade surveillance are more demanding than ever. This is a result of the ever-evolving market abuse tactics being detected and which need to be prevented. For firms, this increased complexity means there’s a need to adopt a consolidated solution that delivers accurate insights when it’s most valuable – at scale both historically and in real time, enabling users to analyze data at a breadth and scale that was previously inaccessible to them.
The flexibility of a high-performance streaming analytics platform will be a game-changer for real time intervention where necessary (e.g. monitoring of high frequency algos) and the timely flagging of abnormal behavior based on large amounts of historical data. Through such technology, firms can become proactive in their response to abnormal behavior in real-time, as opposed to reacting when it is too late. By doing so, firms can work to improve detection rates, as well as make significant savings through fewer false positive cases and ensure operational efficiency is met.